How do we represent space in the brain? Neuroscientists have been working to understand this question since the mid-20th century, when researchers like EC Tolman started experimenting with rats in mazes. When placed in a maze with a food reward that the rats had been trained to retrieve, the rats consistently chose the shortest path to the reward, even if they hadn’t practiced that path before.

Over 50 years later, researchers like Sam Gershman, PhD, of Harvard’s Gershman Lab are still working to understand how our brains encode information about space.
Gershman’s research questions center around the concept of a cognitive map, which allows the brain to represent landmarks in space and the distance between them. He spoke at a Center for Cognitive Neuroscience colloquium at Duke on Feb. 7.
Maps are formed via reinforcement learning, which involves predicting and maximizing future reward. When an individual is faced with problems that have multiple steps, they can do this by relying on previously learned predictions about the future, a method called successor representation (SR), which would suggest that the maps we hold in our brain are predictive rather than retroactive.
One specific region implicated in representations of physical space is the hippocampus, with hippocampal place cell activity corresponding to positions in physical space. In one study, Gershman found, as rats move through space, that place field activity corresponding to physical location in space skews opposite of the direction of travel; in other words, activity reflects both where the rodent currently is and where it just was. This pattern suggests encoding of information that will be useful for future travel through the same terrain: in Gershman’s words, “As you repeatedly traverse the linear track, the locations behind you now become predictive of where you are going to be in the future.”
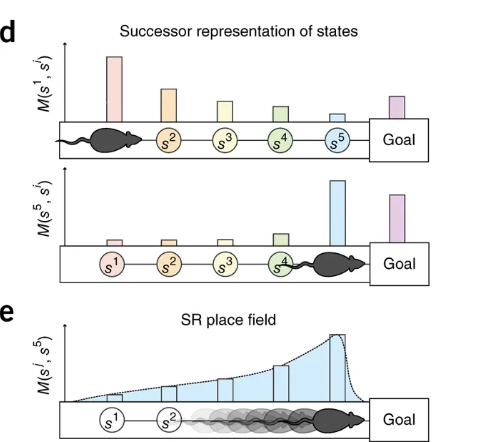
This idea that cognitive activity during learning reflects construction of a predictive map is further supported by studies where the rodents encounter novel barriers. After being trained to retrieve a reward from a particular location, introducing a barrier along this known path leads to increased place cell activity as they get closer to the barrier; the animal is updating its predictive map to account for the novel obstacle.
This model also explains a concept called context preexposure facilitation effect, seen when animals are introduced to a new environment and subsequently exposed to a mild electrical shock. Animals who spend more time in the new environment before receiving the shock show a stronger fear response upon subsequent exposures to the box than those that receive a shock immediately in the new environment. Gershman attributes this observation to the time it takes the animal to construct its predictive map of the new environment; if the animal is shocked before it can construct its predictive map, it may be less able to generalize the fear response to the new environment.
With this understanding of cognitive maps, Gershman presents a compelling and far-reaching model to explain how we encode information about our environments to aid us in future tasks and decision making.