Artificial intelligence has not only inherited many of the strongest capabilities of the human brain, but it has also proven to use them more efficiently and effectively. Object recognition, map navigation, and speech translation are just a few of the many skills that modern AI programs have mastered, and the list will not stop growing anytime soon.
Unfortunately, AI has also magnified one of humanity’s least desirable traits: bias. In recent years, algorithms influenced by bias have often caused more problems than they sought to fix.
When Google’s image recognition AI was found to be classifying some Black people as gorillas in 2015, the only consolation for those affected was that AI is improving at a rapid pace, and thus, incidents of bias would hopefully begin to disappear. Six years later, when Facebook’s AI made virtually the exact same mistake by labeling a video of Black men as “primates,” both tech fanatics and casual observers could see a fundamental flaw in the industry.

On November 17th, 2021, two hundred Duke Alumni living in all corners of the world – from Pittsburgh to Istanbul and everywhere in between – assembled virtually to learn about the future of algorithms, AI, and bias. The webinar, which was hosted by the Duke Alumni Association’s Forever Learning Institute, gave four esteemed Duke professors a chance to discuss their view of bias in the artificial intelligence world.
Dr. Stacy Tantum, Bell-Rhodes Associate Professor of the Practice of Electrical and Computer Engineering, was the first to mention the instances of racial bias in image classification systems. According to Tantum, early facial recognition did not work well for people of darker skin tones because the underlying training data – observations that inform the model’s learning process – did not have a broad representation of all skin tones. She further echoed the importance of model transparency, noting that if an engineer treats an AI as a “black box” – or a decision-making process that does not need to be explained – then they cannot reasonably assert that the AI is unbiased.
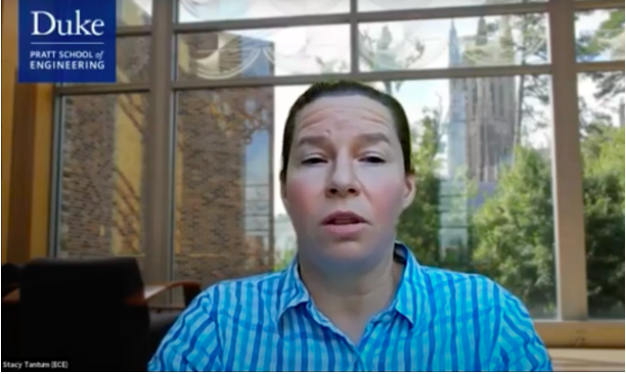
While Tantum emphasized the importance of supervision of algorithm generation, Dr. David Hoffman – Steed Family Professor of the Practice of Cybersecurity Policy at the Sanford School of Public Policy – explained the integration of algorithm explainability and privacy. He pointed to the emergence of regulatory legislation in other countries that ensure restrictions, accountability, and supervision of personal data in cybersecurity applications. Said Hoffman, “If we can’t answer the privacy question, we can’t put appropriate controls and protections in place.”
To discuss the implications of blurry privacy regulations, Dr. Manju Puri – J.B. Fuqua Professor of Finance at the Fuqua School of Business – discussed how the big data feeding modern AI algorithms impact each person’s digital footprint. Puri noted that data about a person’s phone usage patterns can be used by banks to decide whether that person should receive a loan. “People who call their mother every day tend to default less, and people who walk the same path every day tend to default less.” She contends that the biggest question is how to behave in a digital world where every action can be used against us.
Dr. Philip Napoli has observed behaviors in the digital world for several years as James R. Shepley Professor of Public Policy at the Sanford School, specifically focusing on self-reinforcing cycles of social media algorithms. He contends that Facebook’s algorithms, in particular, reward content that gets people angry, which motivates news organizations and political parties to post galvanizing content that will swoop through the feeds of millions. His work shows that AI algorithms can not only impact the behaviors of individuals, but also massive organizations.
At the end of the panel, there was one firm point of agreement between all speakers: AI is tremendously powerful. Hoffman even contended that there is a risk associated with not using artificial intelligence, which has proven to be a revolutionary tool in healthcare, finance, and security, among other fields. However, while proven to be immensely impactful, AI is not guaranteed to have a positive impact in all use cases – rather, as shown by failed image recognition platforms and racist healthcare algorithms that impacted millions of Black people, AI can be incredibly harmful.
Thus, while many in the AI community dream of a world where algorithms can be an unquestionable force for good, the underlying technology has a long way to go. What stands between the status quo and that idealistic future is not more data or more code, but less bias in data and code.

Post by Shariar Vaez-Ghaemi, Class of 2025